Learn how blockchain truly works, master key definitions, and uncover what makes smart contracts so "smart." Dive into the fundamentals, gain valuable insights, and start your blockchain journey today!
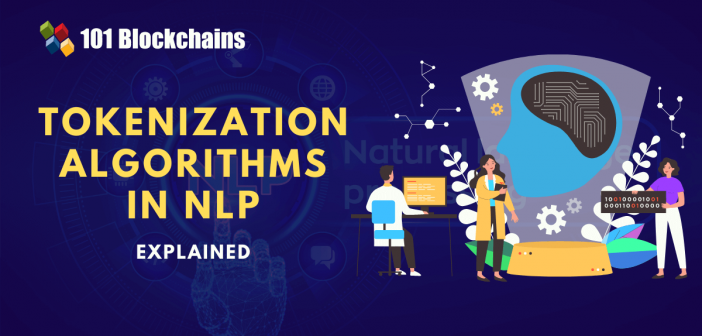
- Guides
Gwyneth Iredale
- on August 24, 2021
An Overview of Tokenization Algorithms in NLP
Language is one of the fundamental aspects responsible for setting the foundations of human civilization. However, gaining fluency in a new language from ground zero can be quite a challenging task. You would have many layers and syntaxes to understand before you master a completely new language. The same is applicable in the case of machines. If you want machines to understand any particular text, then you should divide the word in such a way that machines can understand it. This is where you should look for the significance of the tokenization NLP relationship.
In simple words, it is practically difficult for machines to work with text data without tokenization. Furthermore, tokenization not only breaks down the text data but also plays a crucial role in management of text data. The following discussion offers a detailed overview of different tokenization natural language processing algorithms along with an impression of challenges that you can face in NLP tokenization.
Want to learn the fundamentals of tokenization and its practical implications? Enroll Now: Tokenization Fundamentals Course
Understanding Tokenization
Before starting a discussion on what is tokenization in NLP, it is important to find what tokenization is. Tokenization refers to the process of transforming plaintext data into a string of characters known as tokens. The value of the token will be mapped to the related plaintext data, and the mappings are stored in a token vault or database. It is completely impossible to reverse the token for obtaining original values, and tokenization has found many promising applications in transforming the management and security of data and assets. So, where do you find the tokenization NLP connection?
As a matter of fact, tokenization is one of the foremost steps in natural language processing. It involves the division of a text sequence into different units with relevant individual semantic meaning. The different units are known as tokens. Interestingly, the difficulty of the tokenization process depends on finding the ideal split to ensure that all tokens in the text present the correct meaning.
In addition, it is also important to ensure that the text does not have any tokens excluded from consideration. In order to understand ‘why tokenization is important in NLP,’ you must begin with the fact that text in many languages includes words separated by whitespace. In this case, each word is associated with a semantic meaning.
Excited to know more about tokenization? Read here Everything You Need To Know About Tokenization
Importance of Tokenization for NLP
The first thought that comes to mind when thinking of tokenization in the case of NLP is the unfeasible nature of the idea. With a bunch of text and a computer for processing the text, it is important to wonder about the reasons for breaking the text down into smaller tokens. As a matter of fact, misinformation could be one of the foremost challenges in tokenization in NLP. With a detailed impression of the reasons behind the adoption of tokenization for various NLP use cases, one could find the true value advantages of tokenization.
Tokens are basically the fundamental blocks in natural language. Interestingly, the commonly preferred approach for processing raw text is realized at the token level. The example of Transformer-based models such as the State of The Art (SOTA) Deep Learning architectures for NLP shows processing of raw text at token level. In addition, many other deep learning architectures for NLP, such as LSTM, RNN, and GRU, also have the capabilities for processing raw text at token level.
The tokenization natural language processing link is quite prominently evident since tokenization is the initial step in modeling text data. Users have to carry out tokenization on the text for obtaining tokens. Then, the separate tokens help in preparation of a vocabulary referring to a set of unique tokens in the text.
It is also important to note that you can construct the vocabulary by taking each distinct token in the text into account. Another prolific approach for creating the vocabulary refers to consideration of the top ‘K’ number of frequently occurring words. The vocabulary created through tokenization is useful in traditional and advanced deep learning-based NLP approaches.
- The traditional NLP approaches like TF-IDF leverage vocabulary as features, with each word in the vocabulary perceived as a unique feature.
- In the case of advanced deep learning-based NLP systems, vocabulary can help in developing tokenized input sentences. Subsequently, the tokens for such sentences could work as inputs for the model.
Become a member now to watch On-demand Webinar on Why Tokenization Is The Future!
Algorithms for Tokenization in NLP
The next important aspect in this discussion would refer to the actual agenda, i.e., tokenization algorithm. The algorithm is essential for transforming the plaintext into tokens, and considering the importance of tokenization, it is important to find different algorithms for tokenization in different use cases. Here is an outline of the different types of tokenization algorithms commonly used in NLP.
-
Word Level Tokenization
The first and most common entry among tokenization NLP algorithms refers to word-level tokenization. Word-level tokenization involves the division of a sentence with punctuation marks and whitespace. You could find many libraries in the Python programming language for division of the sentence. The libraries include Keras, NLTK, Gensim, or SpaCy. In addition, users also have the flexibility of using a custom Regex for converting plaintext into tokens.
The division on whitespace could also result in splitting an element that must be considered as a single token. You can encounter profound setbacks as a result of most common issues in names, compounds written as multiple words, and borrowed foreign phrases. Word level tokenization also leads to setbacks, such as the massive size of the vocabulary.
The massive vocabulary size can be responsible for creating performance and memory issues at later stages. In order to address the large vocabulary challenges in tokenization in NLP, an alternative approach for token creation with characters rather than words becomes favorable.
Build your identity as a certified blockchain expert with 101 Blockchains’ Blockchain Certifications designed to provide enhanced career prospects.
-
Character Level Tokenization
Character level tokenization came into existence in 2015 by splitting a text into characters rather than splitting it into words. For example, better would become b-e-t-t-e-r with this tokenization natural language processing algorithm. As a result, you can witness a profound reduction in the vocabulary size to 26 characters for English alongside special characters.
Character level tokenization could also help in better management of misspellings or rare words. The tokenization of text sequences into characters can show promisingly positive results. For example, character-level NLP tokenization models could also help in capturing semantic properties of text effectively.
Although the application of a character-level tokenization algorithm could reduce vocabulary size, you could have a longer tokenized sequence. With the splitting of each world into all characters, the tokenized sequence can easily exceed the original text in length. Furthermore, character-level tokenization does not address the fundamental goal of tokenization as characters alone do not have semantic meaning.
-
Subword Level Tokenization
The challenges in tokenization in NLP with word-level and character-level tokenization ultimately bring subword-level tokenization as an alternative. With subword level tokenization, you wouldn’t have to transform many of the common words. On the other hand, you can just work on rare decomposing words in comprehensible subword units.
For example, if ‘unworldly’ has been classified as a rare word, you can break it as ‘un-world-ly’ with each unit having a definite meaning. In this case, you can find that ‘un’ means opposite, ‘world’ implies towards a noun, and ‘ly’ transforms the word into an adverb. However, subword level tokenization also presents challenges in the approach for dividing the text.
-
BPE Algorithm
Another top example of a tokenization algorithm used for NLP refers to BPE or Byte Pair Encoding. BPE first came into the limelight in 2015 and ensures merging of commonly occurring characters or character sequences repetitively. The following steps can provide a clear impression of how the BPE algorithm works for tokenization in NLP.
- Start with a large enough text
- Develop the definition of a subword vocabulary size
- Divide the word into character sequence and attach a special token to it for showcasing beginning-of-word and end-of-word attributes
- Determine the pairs of sentences in the plaintext along with their frequencies
- Create a new subword on the basis of sentence pairs occurring frequently
- You can repeat division of word into character sequences until you reach desired subword vocabulary size
However, BPE is incapable of offering multiple segmentations as it is a deterministic and input-intensive algorithm. As a result, you would find the same tokenized text for a specific text in all cases.
We have an insightful webinar for you to learn Why Tokenization Is The Future. Become A Member now to watch this on-demand webinar!
Bottom Line
As you can notice clearly, tokenization has profound significance in the domain of natural language processing. Simply put, tokenization is one of the fundamental requirements in natural language processing. The choice of a suitable tokenization NLP algorithm could help in addressing many conventional issues in natural language processing. On the other hand, it is clearly evident that each algorithm fits the requirements of different use cases.
However, implementation of tokenization in blockchain is hard, especially when you bring the vocabulary size into the equation. In addition, it is also important to ensure that the meaning of the token is relevant to the context. Above everything else, tokenization provides a reliable approach for managing text data once you familiarize with it. Learn more about tokenization and how it can be used for NLP right now!