Learn how blockchain truly works, master key definitions, and uncover what makes smart contracts so "smart." Dive into the fundamentals, gain valuable insights, and start your blockchain journey today!
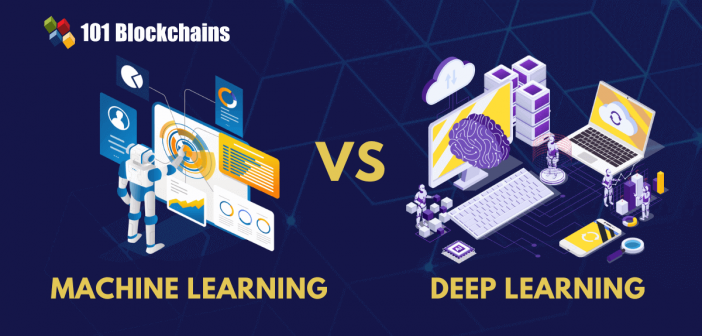
- Comparisons
James Howell
- on May 24, 2024
Deep Learning vs. Machine Learning: Key Differences
Digital technologies such as Deep Learning and Machine Learning have taken the world by storm. Although these technologies are in their nascent stage, they have shown immense potential. The emergence of these technological concepts is a boon for individuals and businesses alike. Today, it has become a necessity to gain insight into the technologies that are revolutionizing our lives.
However, if you want to understand these technologies at a comprehensive level, you need to familiarize yourself with not only the unique concepts but also the differences that exist between them. For someone who wants to have a promising career in the competitive IT industry, it is essential to know how concepts such as DL and ML are influencing the trajectory of AI. Before understanding the deep learning vs machine learning difference, it is essential to have a clear knowledge of the respective technological concepts.
What is Machine Learning?
Machine Learning (ML) is a subdomain of Artificial Intelligence technology. ML primarily focuses on the creation of algorithms and statistical models that allow computer systems to learn. Thanks to ML, computers can make decisions or predictions without the need to explicitly program them.
Thus, Machine Learning involves training algorithms to support the identification of relationships and patterns. Ultimately, such patterns help make predictions about new data. In simple words, ML is the type of Artificial Intelligence that relies on minimum human interference.
Some of the chief features of Machine Learning are:
- ML algorithms enable the automation of processes, which help in locating patterns in data.
- Machine Learning techniques are ideal for processing big data as they can handle a substantial volume of data.
- Innovative technology can help to make predictions about future events.
- ML algorithms are capable of discovering wide patterns in data that can help analyze unexplored and fresh data.
Take your first step towards learning about artificial intelligence through AI Flashcards
What is Deep Learning?
Deep Learning is a part of Machine Learning. It makes use of neural networks with diverse layers to analyze complex associations and patterns in data. It is capable of mimicking the human brain to carry out a broad range of tasks, such as speech recognition as well as natural language processing. One of the main highlights of Deep Learning models is that they are capable of learning. Hence, they can improve over time, and their level of accuracy can improve as they process data.
Some of the chief features of Deep Learning are:
- The Deep Learning technology works by mimicking the human brain.
- The deep neural networks that makeup DL have numerous layers that support the model in learning the data’s hierarchical representation.
- The features of DL relating to high power and flexibility make it suitable for varying tasks like speech and image recognition, etc.
- Deep Learning algorithms are highly helpful when there are a large number of inputs as well as outputs.
Although to a layman, the terms Artificial Intelligence, Machine Learning, and Deep Learning may sound similar. The reality is that each of these concepts is different from one another. However, they are related to each other. Now that you have clarity on deep learning and machine learning concepts, it is time to answer the following question: What are the differences between deep learning and machine learning?
Excited to learn the fundamentals of AI applications in business? Enroll now in the AI For Business Course
Difference between Deep Learning and Machine Learning
There exist a number of deep learning vs machine learning differences. By identifying how the technological concepts are unique, it is possible to identify their true potential.
-
Conceptual difference
Machine Learning is the branch of Artificial Intelligence. Since it is trained on statistical models and algorithms, it is capable of making predictions. The technology relies on historical data as well as training to strengthen its capabilities. A key thing to bear in mind is that ML relies on human intervention so that data can be fed and improvements can be made.
Deep Learning can be defined as the subset of Machine Learning technology. It is a model that can help an algorithm ascertain whether a prediction is accurate or not. The role of neural networks is indispensable in Deep Learning. As neural networks empower software to comprehend complex patterns, there is no need for human intervention.
-
Data Representation
The difference between machine learning and deep learning arises from the use of data representation. In the case of machine learning, the data representation that is used is quite different from that of deep learning. In Machine Learning, structured data is used. This type of data is highly valuable since it can be searched and analyzed in a simple and efficient manner. ML techniques may find it challenging to work on unstructured data. This is due to the fact that the training dataset may have infinite variations.
The data representation that is used in Deep Learning is different as it involves neural networks. DL is capable of working with not only structured data but also with unstructured data. The technology can be used for a broad range of unstructured data such as images, audio, text as well as tables containing data.
-
Data points
The values that are arranged in structures are known as data points. Machine Learning encompasses thousands of data points, whereas Deep Learning involves millions of data points. This feature goes on to show that Machine Learning is capable of working well with relatively small databases. On the other hand, Deep Learning relies on a humongous volume of data while carrying out its task. DL basically requires large amounts of data so that it can understand and perform tasks better than conventional ML algorithms.
-
Training time
While exploring deep learning vs machine learning, a key difference that arises is related to the training time. Training time is basically the time that is taken by a model for the purpose of training on a dataset. When it comes to ML algorithms, they require less time for training. The training time may range between a few seconds to a number of hours. However, the same is not the case when it comes to Deep Learning. The training time for a Deep Learning neural network may span between a few hours to several weeks. However, it may be possible to adopt certain methods to hasten the training time.
-
Effectiveness and setting up time
When it comes to Machine Learning, it may be possible to set up and run the system in no time. However, although it may be easy to set up the system, it does not mean that its effectiveness is guaranteed. In fact, the effectiveness of the system may be hampered. On the contrary, setting up Deep Learning may seem time-consuming, but there is no compromise on the effectiveness of the system. Once the system is up and running, there is a need for limited or no intervention. It is capable of generating results in an effective manner. Moreover, the quality of the outcome is likely to get better with the passage of time.
-
Need for resources
The resources that are required for ML systems and DL systems may vary considerably. It is possible to execute Machine Learning on simple and standard computer systems. As ML does not require a large volume of computational power, it can be supported by a standard computer. However, the same cannot be said about deep Learning.
Deep Learning systems require powerful hardware and software resources so that they can function properly. As DL involves a broad number of operations, it needs a robust system that can support it. In the absence of appropriate resources, the efficiency and functionality of DL may be diminished.
Want to understand the importance of ethics in AI, ethical frameworks, principles, and challenges? Enroll now in the Ethics Of Artificial Intelligence (AI) Course
-
Featurization process
When it comes to deep learning vs machine learning, a key difference is related to the featurization process. In machine learning, it is essential for users to identify and create features accurately. It shows that the technology relies on users to carry out its tasks and functions. However, in the case of deep learning technology, there is no need for users to intervene. DL is capable of learning high-level features from the data itself. That’s not all! Deep Learning can create new features all by itself without relying on the help and support of humans.
The table below presents a comparison between Deep Learning and Machine Learning.
<pre>Please include attribution to 101blockchains.com with this graphic. <a href='https://101blockchains.com/blockchain-infographics/'> <img src='https://101blockchains.com/wp-content/uploads/2024/05/Deep-Learning-VS-Machine-Learning-1.png' alt='deep learning vs machine learning='0' /> </a>
Future of ML and DL
In the technology-driven era, the future of Machine Learning and Deep Learning is full of promise and potential. The unique features and capabilities of these technologies make them highly capable of carrying out a broad range of tasks and operations. As AI technology is reaching new heights, the future of both ML and DL is extremely bright.
If you wish to make your career in the dynamic IT domain, it is a must to broaden your knowledge about AI, ML, and DL. Currently, these technologies are serving as catalysts and influencing the trajectory of the IT industry. The emergence of technologies such as Machine Learning and Deep Learning have given rise to new job opportunities. If you are passionate about establishing yourself as a competent IT professional, you need to brush up on your skills relating to ML and DL.
Identify new ways to leverage the full potential of generative AI in business use cases and become an expert in generative AI technologies with Generative AI Skill Path
Conclusion
In the Artificial Intelligence landscape, technologies such as Machine Learning and Deep Learning play a key role. The key differences between ML and DL have been presented. The technologies give a glimpse into the power of modern technologies. There exist a number of deep learning vs machine learning differences relating to the underlying concepts, data representation, use of data points, training time, effectiveness, and setting up time resource requirements and featurization processes.
Today, both machine learning and deep learning are revolutionary technologies. The emergence of these technologies has been of immense value. The future of these technological concepts is full of high potential. These modern technologies have given rise to new opportunities and possibilities in the vast IT industry.